Article
Deconstruct Store Performances to Improve the Planning Process
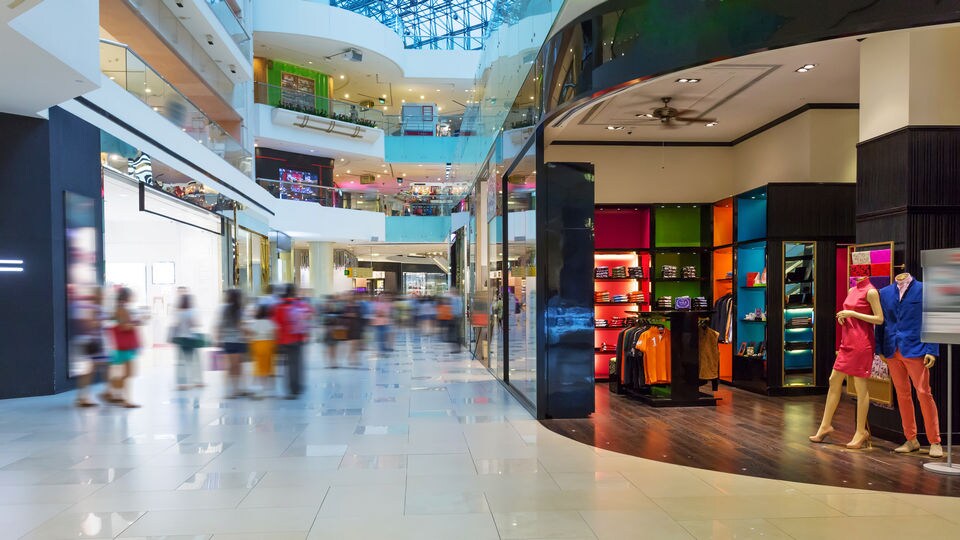
Going back to my planning days working directly in retail, I had a boss who reviewed all sales plans prior to distribution to stores. I had been given an overall sales number to hit, with little regard to the actual year-over-year (comp) performance.
I took this as an opportunity to socialize the value of traffic in planning. The plans that I presented to my boss had a very wide range of sales comp expectations that were driven by the reality of each store’s traffic base. As she reviewed the plans, she kept stopping on stores that I had planned with negative comps. Why had I planned them in this manner, she wanted to know. I showed her the traffic comps for all the negative sales comp stores. In every case, each store was down at least -10% in traffic, although I had planned their sales higher than that.
Her first reaction was that this was not acceptable. My boss never planned any store in the negative and was not going to start now. She wanted me to go back and make them all positive or at least flat to the prior year. Assuming this was going to happen, I had also brought a second draft, with no stores in the negative yet with the same overall sales target. The problem with this approach was that stores with currently higher trends were going to get lower plans than their current trends called for. And the negative comp stores would get plans that they certainly would never achieve. This was simply a math exercise that could provide a sales plan number, but without any rigor in determining how each store’s current and historical performance would support it.
Using this second draft meant that the currently negative comp stores would get sales plans that they couldn’t achieve and since there were paid bonuses for making their plans, they would already be demoralized and would not even try to succeed. Conversely, the stores with unrealistically easy plans would not work hard to achieve even higher results because they did not have to. Or worse still, they would all hit the easy targets and all bonus unrealistically. Net effect: we would have lost the plan before we even started, and we might pay out unwarranted sales bonuses.
The other point to make is about those negative comp plan stores. As I mentioned, each of them had traffic at -10% or worse. Since in most retail organizations, store teams do not drive traffic (typically, it’s the location and marketing), teams with bad traffic comps would not bonus due to circumstances beyond their control. And if there are stores with poor traffic comps, there were also stores with exceptional traffic comps that were succeeding simply because of the traffic increases. Using the second draft, we would be rewarding those stores even if they did not contribute to the overall sales comps. This is not a recipe for revenue and margin success.
“We agreed to start sales plan assumptions by quantifying each store’s traffic trend and we introduced a new metric to the company: Sales per Shopper (SPS). Year-over-year SPS distills the traffic and sales comp review down to one metric.”
After reviewing both sets of plans and more discussion on the topic, my boss reversed course and we set plans differently. We looked at both trended Sales and Traffic performance to ensure that we were recognizing those performers who were making the best of a bad situation (traffic comps were lower than sales comps) and challenging those stores that were coasting along with little contribution (traffic comps were higher than sales comps). We agreed to start sales plan assumptions by quantifying each store’s traffic trend and we introduced a new metric to the company: Sales per Shopper (SPS). Year-over-year SPS distills the traffic and sales comp review down to one metric. Either the store is doing everything it can to maximize value, or it is not. Let’s look at a couple of real examples using performance metrics to determine each store’s unique contribution to the overall goal by deconstructing their sales results (these are actual stores’ data).
Store | Avg. Weekly Sales |
Sales per Shopper |
Average Transaction Size |
Conversion | Average Weekly Traffic |
Labor Hours | STAR |
---|---|---|---|---|---|---|---|
Store #1 | $18,345 | ||||||
Store #2 | $17,186 |
In the first view, Store #1 is the better store because they have the higher sales. But let’s look a little further.
Reviewing the two stores’ performance metrics, Store #1 ranks lower in these more complex metrics except Average Transaction Size. More importantly, they don’t’ appear to be making the most out of their opportunities because their Sales per Shopper (how much is each customer who walks in the store worth?) is over a dollar worse than the other store. Remember that we said that SPS is within the store’s control while traffic is mostly outside of their control? This would mean that in comparison to Store #2, Store #1 is underperforming.
Store | Avg. Weekly Sales |
Sales per Shopper |
Average Transaction Size |
Conversion | Average Weekly Traffic |
Labor Hours | STAR |
---|---|---|---|---|---|---|---|
Store #1 | $18,345 | $3.93 | $33.30 | 11.8% | |||
Store #2 | $17,186 | $5.17 | $30.40 | 17.0% |
This underperformance is borne out when you consider that they have more traffic. By over 1,300 compared to Store #2! Yet they only have roughly $1,200 more in sales. When you look at the results in this manner, the best you might say is that it’s a tie between the stores, with one working harder and one benefiting from a higher traffic base. But what does the coverage tell you about the service level in each store and how it may be impacting each store’s overall performance?
Store | Avg. Weekly Sales |
Sales per Shopper |
Average Transaction Size |
Conversion | Average Weekly Traffic |
Labor Hours | STAR |
---|---|---|---|---|---|---|---|
Store #1 | $18,345 | $3.93 | $33.30 | 11.8% | 4,668 | ||
Store #2 | $17,186 | $5.17 | $30.40 | 17.0% | 3,326 |
Revealing the labor hours, you can see that Store #1, due to its sales results, benefits from additional labor hours – 81 more than Store #2. On the surface, you would think that this is a decided advantage and as a result, Store #1 should easily outperform the other store. And yet, they do not. The reason may lie in the Shopper to Associate Ratio (STAR). This metric takes the traffic count and divides it by the labor hours to determine what the service level is. In this case, that ratio for Store #1 is 14.0, which means that they have one associate available for every 14 shoppers who walk in the store. This is unfavorable to Store #2’s 13.2. The disparity might explain the big difference between Sales per Shopper results between Store #1 and Store #2.
Store | Avg. Weekly Sales |
Sales per Shopper |
Average Transaction Size |
Conversion | Average Weekly Traffic |
Labor Hours | STAR |
---|---|---|---|---|---|---|---|
Store #1 | $18,345 | $3.93 | $33.30 | 11.8% | 4,668 | 333 | 14.0 |
Store #2 | $17,186 | $5.17 | $30.40 | 17.0% | 3,326 | 252 | 13.2 |
This type of deconstruction helps to inform sales planning and can be a very valuable method for getting at the root cause of performance breakdowns. For example, what if we took these results and added in data points on inventory out-of-stocks or shopper disappointment %? Could this help to explain lagging conversion rates or underperforming average transaction sizes? Linked to labor allocation, is there a direct relationship between coverage and store execution?
All good things – and even some bad things – happen inside stores. Planning without considering all the moving parts at this most granular level risks limiting revenue and profit opportunities at the top level.
“This type of deconstruction helps to inform sales planning and can be a very valuable method for getting at the root cause of performance breakdowns.”
About Brian Field
Brian Field oversees the Global Retail Consulting Practice as senior director at Sensormatic Solutions. He provides retailers with insights that drive revenue while controlling the bottom line. Prior to joining Sensormatic Solutions, Brian spent the better part of four decades working directly in retail. His experience includes strategic planning and analysis, direction of store finance and store operations, store systems integration and project management, store sales and management, training design and merchandising. Brian’s retail sector experience includes Specialty Apparel, Consumer Electronics and Housewares for companies as diverse as Chico’s FAS, Circuit City, and Macy’s.
Explore Related Topics
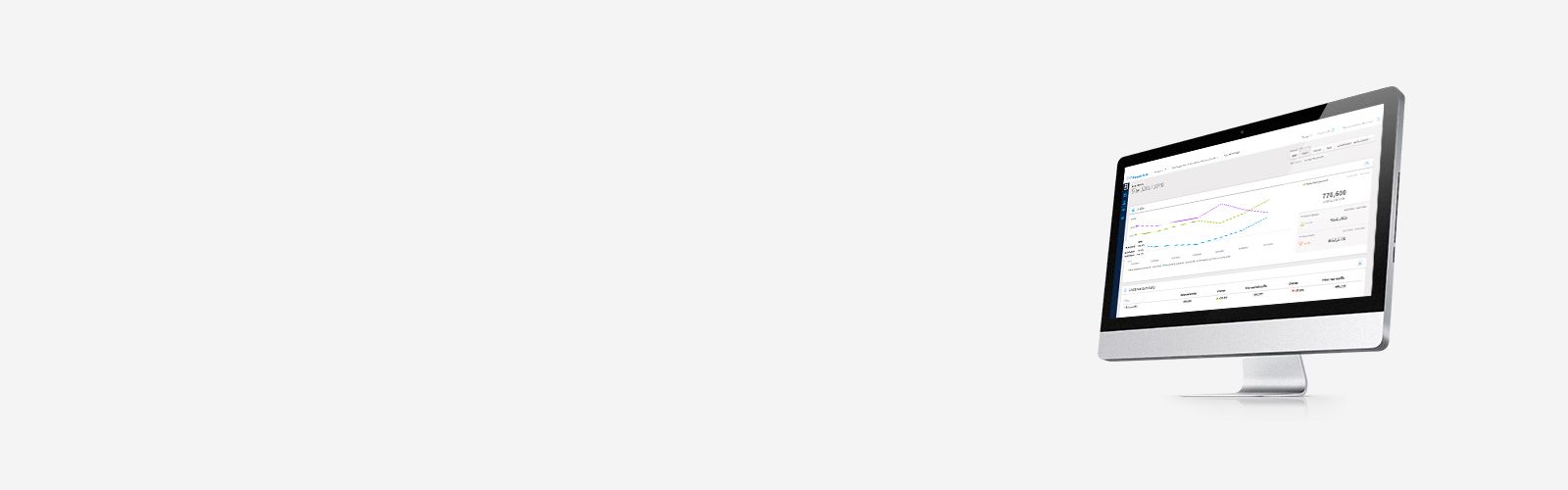